In this article…
xG (expected goals) is an increasingly popular statistic in football to measure the quality of chances a team creates in a game.
You will often see an xGF (expected goals for) and xGA (expected goals against) value when looking through the statistics of a match and may have questioned what these statistics are measuring, and how you can use it to your advantage when betting.
Thinking of the metric as a score for ‘chance quality’ rather than ‘expected goals’ can make it simpler to understand.
This article will break down what xGF and xGA are and how they are calculated to give more context to these unique measurements.
What is xG?
In simple terms, xGF is how many goals a team is likely to score in a game by considering the quality of chances created. This is done by measuring the probability of each shot taken by a team and giving it an xG value which represents the probability of it resulting in a goal, for example a shot from distance could generate an xG of 0.01 – suggesting the shot had a 1% chance of going in.
The total xG you see at the end of the game is the sum of the xG of all the shots taken. xGA is the opposite, it stands for expected goals against and is how many goals a team is likely to concede based on the quality of chances they allow the other team to have on their goal.
For example, in England’s 0-0 draw with Slovenia at Euro 2024: Slovenia posted 0.15 xGF. This figure came from their four shots that were worth 0.07, 0.02, 0.03 and 0.03 xG individually, their total xGF for the match is the sum of them – 0.07 + 0.02 + 0.03 + 0.03 = 0.15. England’s xGA (expected goals against) figure for the match, therefore, was 0.15.

Teams will end up with an xGF and an xGA at the end of a game which can give us a more accurate representation of the quality of chances a team creates or concedes.
This is particularly useful in a low-scoring sport like football as the result isn’t always an indicator of performance. For example, Romania beat Ukraine 3-0 in their Euro 2024 opener, however, the xG data suggested that the game was closer than the scoreline portrays. Romania scored three goals from nine shots and posted a total of 1.20 xGF, whereas Ukraine did not score from 13 shots and 0.75 xGF. 3 goals difference in the scoreline, but only 0.45 in terms of xG.
What came in the rest of the group stage supported the notion that the teams were more closely matched than the 3-0 scoreline suggested, as they both finished the group stage on four points.
It’s important to remember that xG only focuses on shots, it doesn’t matter how threatening a counter-attack or sequence of play is, possession and territory is not included in xG, if there isn’t a shot at the end of the attack it won’t count towards xG.
It’s important to look at where the expected goals are coming from, especially if penalties take place in the match as they inflate the statistic.
It differs slightly per stats provider, but a penalty (according to Opta) has an xG value of 0.79. Therefore, from a betting perspective, it can be useful to look at open play xG or non-penalty xG to avoid data being skewed.
Context is everything, the xG scoreline in a match may end 2.10 – 0.68. But if the team with 2.10 xGF had two penalties in the game, 1.78 xG from two shots, they only generated 0.32 aside from those two incidents, and the xG scoreline would have been slightly misleading in terms of their dominance of the game had you not known about the penalties.
Furthermore, xG isn’t just used to measure a team’s output, because each shot is tracked and it generates an xG for specific players which can hold real value in certain markets such as anytime goalscorer.
An individual player’s xG will be the sum of the xG values of the shots they had in the game.
For example, in Ukraine’s final group game of Euro 2024 against Belgium (0-0), Artem Dovbyk had three shots. The first was a 0.06 xG shot, the second was 0.09 and the third was 0.07. Dovbyk’s player xG for the match was 0.22 (0.06 + 0.09 + 0.07).
If you want to back an anytime goalscorer, it is useful to know if they have been getting on the end of high-quality goalscoring opportunities recently or not. Their xG will offer an insight into that.
How is xG calculated?
According to StatsBomb:
“Each xG model has its own characteristics, but these are the main factors that have traditionally been fed into the large majority of Expected Goals models: distance to goal, angle to goal, body part with which the shot was taken, and type of assist or previous action (through ball, cross, set-piece, dribble, etc…). Based on historical information of shots with similar characteristics, the xG model then attributes a value between 0 and 1 to each shot that expresses the probability of it producing a goal.”
Are Headers & Corners included in xG?
xG is a very detailed measurement, as well as having specific measurements for shots from corners and penalties, it will even go as far as to factor in what part of the body is used for the shot, so a header, for example, would typically be a smaller xG than a shot with a player’s foot from the same position. They work this out by considering the proximity to the goal of the header and the ratio of goals to attempted header statistics from that location.
How does xG help when picking a football bet?
Putting it in the context of football betting, we can use xGF to show us what teams create the highest quality chances. It’s no surprise that Manchester City are up there when it comes to this statistic, but it was Liverpool who generated the highest xG in the Premier League last season with 89.4, scoring 86 goals. Manchester City got the edge over Liverpool by outperforming their xG of only 81.9 to score 96 goals, which indicates that Manchester City were far more clinical in front of goal.

We can also look at sides that underperform their xG, Everton were the biggest culprit of this last season generating an xG of 54.9 but only scoring 40 goals – this suggests their finishing was poor overall. As against the xG algorithm, that has 1000s of shots built into it, they scored almost 15 goals less than if their finishing had been average.
xGF v xGA – Finding Betting Value
In recent years, the bookmakers’ odds have become more xG-based with the metric being heavily factored into the prices that they set. This has made it more difficult to find value using xG, but it is still worth considering before placing a bet as it can help a bettor gain a greater understanding of the recent and long term performance levels of a team.
xGF and xGA tell us a lot about players as well, specifically strikers and goalkeepers. A side that has a highxGA but doesn’t concede a lot of goals could be an indicator of a solid goalkeeper such as Manchester United, who allowed an xGA of 70.1 but only conceded 58 goals indicating that Andre Onana is a very busy goalkeeper, supported by his 3.9 saves per game for the United keeper which was the 4th-highest in the Premier League last season.
Newcastle United created the most big chances in the Premier League last season (134), they generated an xG of 77.1, scoring 85 goals. With the knowledge that they create high-quality chances with regularity and have a striker in Alexander Isak whose goalscoring output was closely aligned to his xG (20.3, 21 goals scored) we could look at backing him as an anytime goalscorer.
Judging goalkeepers using xGOT
According to Stats Perform (more commonly known as Opta):
“xG measures the quality of the chances that a side makes and xGOT builds on this to tell us what a team managed to do with these chances. Essentially, xG is a pre-shot model while xGOT is a post-shot model.
“The Expected Goals on Target model is built on historical on-target shots and includes the original xG of the shot but also the goalmouth location where the shot ended up. It gives more credit to shots that end up in the corners compared with shots that go straight down the middle of the goal. This model is only for on-target shots given that if you don’t get your shot on target, there’s a 0% chance that it will result in a goal.”
“Another use of xGOT is as a tool for analysing goalkeeper performances. We can use it to highlight the goalkeepers who have under-conceded (overperformed) considering the quality of the shots on target they’ve faced. This can be seen by calculating the number of goals prevented (calculated as xGOT conceded from shots on target faced, minus goals conceded).
“This metric allows us to distinguish between the goalkeepers who are making high quality saves and those whose save counts may have been inflated by easy, low quality shots.”
Below is a table of the top ten goalkeepers in the Champions League in 2023/24 according to Goals Prevented.
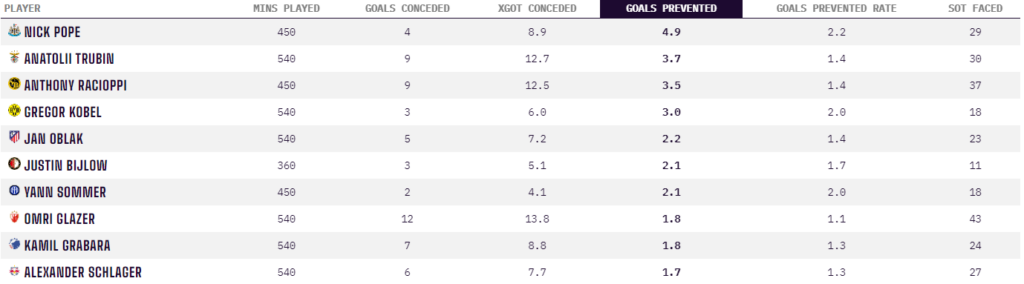
For example, in part due to the complexity of the shot, Jude Bellingham’s overhead kick goal against Slovakia was valued at 0.15 xGF. However, it had an xGOT value of 0.36 (suggesting a much higher probability of it going in) due to the strike going in the corner.
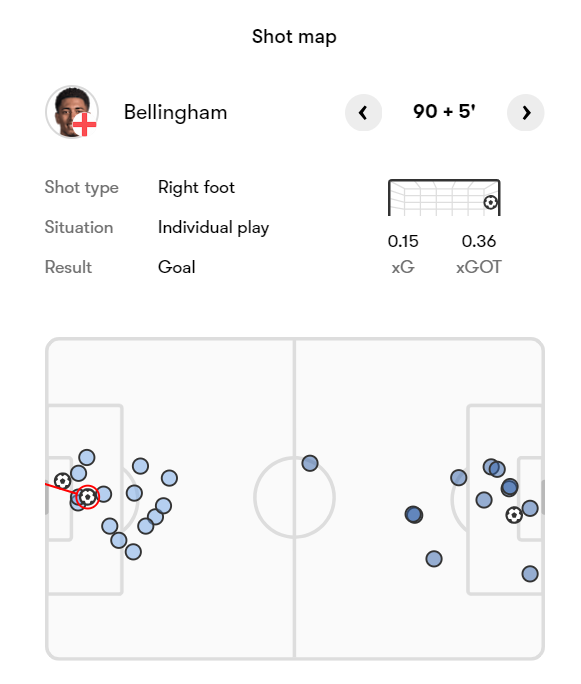
How to use xG when analysing a football match
xG does not consider previous records of a given player, it’s only calculated on the attempts they have during that specific game.
If Brentford’s Neal Maupay and Tottenham Hotspur’s Heung-Min Son have the exact same chance, the xG will be the same, even though the latter is commonly accepted to be a better finisher.
xG doesn’t consider the context of a fixture, it remains the same for every match. For example, if Nottingham Forest were travelling to Anfield to play Liverpool, we would expect them to sit in a low or mid block and look to catch Liverpool out on the counter-attack with the Reds dominating possession.
This means that Nottingham Forest will likely to produce a low xG and a high xGA but doesn’t mean they are going to lose the game. xG and xGA can portray teams that focus on the defensive side of the game as poorer than they are in reality, when it can be a very effective way of managing a side to victory. This can be seen in the poor xG data compared to strong league finishes of managers like Roy Hodgson at Crystal Palace and David Moyes at West Ham United.
It is important to consider game-state when you read into xG numbers. When teams are winning for extended periods of matches their xG data can look worse than a team who are losing for extended periods. This is because the onus is on the side who are losing to push for a goal (and therefore take more shots) than the team that are content holding onto their lead, who often sit back and concede more shooting opportunities as a result.
In summary, xGF and xGA are useful statistics that we can use to inform our selections. It’s important not to take it all at face value and use other statistics in tandem with them to make the most of this metric to select winning bets.
It’s something that is evolving still and will only get more accurate with time and testing, it’s also important not to ignore the context we find xGF and xGA in, consider the playstyle of a team and the quality of individual players when weighing up use of the metric in your betting selections.
Written by an Andy verified content writer
18+ please gamble responsibly.